About
I am a PhD student at Imperial College London under the supervision of Dr. Sergio Maffeis. My research focus is on applying Reinforcement Learning to difficult problems in cyber security Prior to this I received a Masters of Engineering from University College London, earning the ‘Outstanding MEng Graduating Student’ prize from the Department of Electronic and Electrical Engineering.
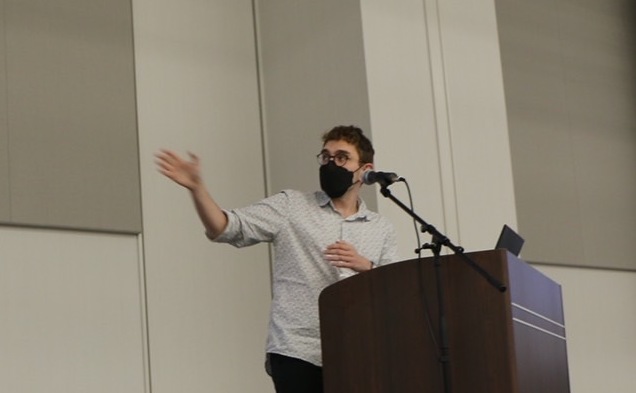
Security & Machine Learning.
Research Interests
- Web Security
- Continual Learning
- Network Defence
- Reinforcement Learning
- Vulnerability Detection
- Adversarial Machine Learning
Skills
Education and Experience
Education
Doctorate of Philosophy
2020 - Present
Department of Computing, Imperial College London
Research focused on developing Reinforcement Learning applications that improve security in web applications (e.g. XSS, SQLi, Command Injection, APIs) and computer networks (autonomous network defence). This research has been presented at IEEE TrustCom (winning best paper), AsiaCCS (winning best poster), and CAMLIS.
Masters of Engineering
2016 - 2020
Electronic Engineering with Computer Science, University College London
Design and implementation of a novel graph-deep-Q-network in Tensorflow. Project lead for team on a computer vision task that developed an advanced driver assistance system to predict lane changes of vehicles. I oversaw the data processing on this task, in addition to the implementation of trajectory prediction algorithm, and model hyper parameter tuning.
Professional Experience
Research Intern
September 2022 - December 2022
IBM Research
Research on using NLP methods to develop privacy and security in Large Language Models (LLMs). Involving: transformers, text classification, and reinforcement learning. This work was used to win the second MLMAC Challenge.
Enrichment Student
November 2021 - Present
Alan Turing Institute
Collaboration for Autonomous Cyber Defence, involving developing techniques to combat the large state space when applying reinforcement learning. Our Hierarchical Reinforcement Learning model went on to win the First CAGE Challenge this international challenge has agents defend a small network against multiple classes of advanced persistent threat. Read about it in our paper that won the best poster award at AsiaCCS '22 here or page 19 of the Alan Turing Institute Annual Report 2021-2022. We also won the Third CAGE Challenge .
Graduate teaching Assistant
October 2020 - Present
Department of Computing, Imperial College London
- Network and Web Security (Spring 2021, Spring 2022, Spring 2023)
- Reinforcement Learning (Winter 2020, Winter 2021)
- Networks and Communications (Spring 2022, Spring 2023)
- Deep Learning (Spring 2023)
- Reasoning About Programs (Spring 2021)
Publications
SQIRL: Grey-Box Detection of SQL Injection Vulnerabilities Using Reinforcement Learning
S. Al Wahaibi, M. Foley, S. Maffeis. SQIRL: Grey-Box Detection of SQL Injection Vulnerabilities Using Reinforcement Learning, Proceedings of the 32nd USENIX Security Symposium, 9-11 August, 2023, Anaheim, USA. [PDF][CODE]
Matching Pairs: Attributing Fine-Tuned Models to their Pre-Trained Large Language Models
M. Foley, A. Rawat, T. Lee, Y. Hou, G. Picco, G. Zizzo. Matching Pairs: Attributing Fine-Tuned Models to their Pre-Trained Large Language Models, Proceedings of the 61st Annual Meeting of the Association for Computational Linguistics , 9-14 July, 2023, Toronto, Canada. [PDF][CODE]
Haxss: Hierarchical Reinforcement Learning for XSS Payload Generation
IEEE Best Student Paper
M. Foley, S. Maffeis. Haxss: Hierarchical Reinforcement Learning for XSS Payload Generation, 2022 IEEE 21th International Conference on Trust, Security and Privacy in Computing and Communications (TrustCom’ 22), 9-11 December, 2022, Wuhan, China. [DOI][CODE]
Inroads in Autonomous Network Defence using Explained Reinforcement Learning.
M. Foley, M. Wang, Z. M, C. Hicks, V. Mavroudis. Inroads in Autonomous Network Defence using Explained Reinforcement Learning. 2022. In Proceedings of the Conference on Applied Machine Learning for Information Security. October 20-21, 2022, Arlington, VA. [SLIDES][VIDEO][CODE][PDF]
Autonomous Network Defence using Reinforcement Learning
Best Poster Award
Myles Foley, Chris Hicks, Kate Highnam, and Vasilios Mavroudis. 2022. POSTER: Autonomous Network Defence using Reinforcement Learning. In Proceedings of the 2022 ACM Asia Conference on Computer and Communications Security (ASIA CCS ’22), May 30–June 3, 2022, Nagasaki, Japan. ACM, New York, NY, USA, 3 pages. [DOI][PDF][CODE]
Talks and Media
-
Hierarchical Reinforcement Learning for Cyber Security [POSTER], Imperial Computing Conference, 1 July 2022
Hacking websites with Reinforcement Learning: an XSS story, Machine Learning Cyber Security Symposium at Imperial College London, 31 May 2022
Reinforcement Learning for Computer Security, Imperial College London Department of Computing PhD showcase, 12 Feburary 2022
Contact
Send me an email, its the best way to contact me!